In recent years, Big Data projects have become an important organizational focus for many companies. Companies collect and store an enormous amount of information from a multitude of different sources that can be leveraged and capitalized on in ways never imagined possible. New technologies have made it cost effective and attainable for even more companies to enter the world of Big Data. The uses and benefits of Big Data technology is as infinite as the imagination, from target marketing for a product or service, spotting fraud, optimizing cancer treatments, to predicting the next big flu outbreak. For many companies Big Data is not a “nice to” to stay competitive. There is no option but to invest in Big Data or be left behind.
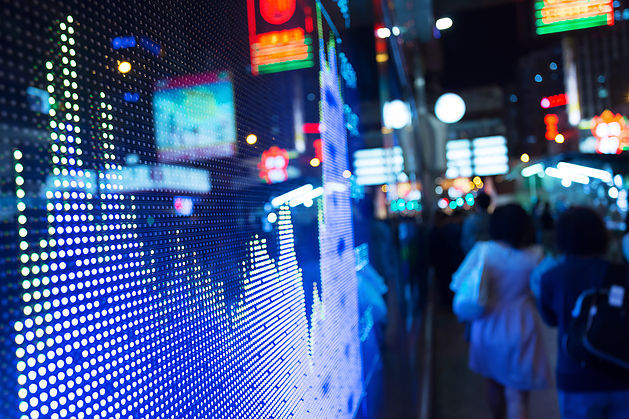
Big Data is becoming big business, an International Data Corp (IDC) report forecasts annual spending on Big Data technology and services will grow at an annual growth rate of 23.1% with annual spending reaching $48.6 billion in 2019. (1) Other studies from some major consultancies and software vendors have even higher projections.
There are big risks as organizations grapple with the legal implications and potential liabilities of Big Data usage. The U.S. Department of Health & Human Services reported an increase in privacy related complaints rising from 12,974 in 2013 to 17,779 in 2014 (2) and the Information Security Media Group’s Data Breach Today Website – Litigation Section (3), highlights the many legal actions related to Big Data technology.
With a high projected level of growth, investment and potential risk, it is important that Big Data projects are well conceived, planned and implemented.
Basics First – What is Big Data?
What is Big Data? Big Data is the term applied to data collections that are so large they require new, advanced and evolving data processing tools, applications and consideration to find, collect, store, secure, clean, manipulate, query, interpret, extract, share or access. (4)
The marketplace defines and references Big Data in the following ways:
IBM has a public website dedicated to Big Data and defines Big Data as follows:
“What is Big Data? Big data is being generated by everything around us at all times. Every digital process and social media exchange produces it. Systems, sensors and mobile devices transmit it. Big data is arriving from multiple sources at an alarming velocity, volume and variety. To extract meaningful value from big data, you need optimal processing power, analytics capabilities and skills.” (5)
Microsoft references Big Data within their public website as follows:
“You need a new kind of data warehouse to handle the exponentially growing volume of data, the variety of semi-structured and unstructured data types, and the velocity of real-time data processing. Microsoft’s modern data warehouse solution integrates your traditional data warehouse with unstructured big data—and it can handle data of all sizes and types, with real-time performance.” (6)
SAP defines Big Data on their public website as follows:
“Big Data is the ocean of information we swim in every day – vast zettabytes (a) of data flowing from our computers, mobile devices, and machine sensors. With the right solutions, organizations can dive into all that data and gain valuable insights that were previously unimaginable.” (7)
Oracle defines Big Data on their public website as follows:
“Big data describes a holistic information management strategy that includes and integrates many new types of data and data management alongside traditional data.” (8)
Big Data Project Tips
Big Data projects are a very unique project type. They are often fast moving and require organizational and project leadership to carefully blend many layers of ever changing technology together, fuzzy business goals and people with many different responsibilities and concerns from many different organizational areas. There are many actions that can be taken to help ensure project success.
Big Data Project Tip #1 – Define what Big Data means
As the definition and quotes illustrate, there is no true Big Data definitional standard. Search on the term “Big Data Definition” and you will see no clear standard. Defining what Big Data means to your organization or project is very important to its success. Although there is a common thread or theme in most definitions related to large amounts of data, the marketplace presents many different variations of what Big Data is and can be, often based on a vendor’s hardware, software or tools.
The starting point for any Big Data project should be to make certain that everyone has an understanding of what Big Data means and will mean. Ensuring all sponsors, stakeholders and project team members agree on what Big Data means to the organization, clarifying and aligning what the goals and objectives of a Big Data project are.
Big Data Project Tip #2 –Don’t confuse the technical part with the business part
Projects often have many different types of actors that must be managed to success. A Big Data project is no different. Carefully define and communicate the roles and responsibilities of team members at the start of a project. Make certain that technical project team members provide technical knowledge and support and business project team members provide business knowledge and support. A little knowledge executed by the wrong set of hands can create big problems ranging from improper hardware and software set up by a well-meaning business person that creates a major security breach to a technical person deciding what data can be used and violating the privacy rights of employees or customers. Just because someone can doesn’t mean they should.
Big Data Project Tip #3 – Don’t assume today is the same as yesterday or tomorrow is the same as today
In Big Data marketplace and Innovation comes quickly and new technologies appear quickly and existing technologies are updated frequently.
Apache Hadoop,the widely used open-source software framework leveraged by many Big Data projects for distributed storage and distributed processing of very large data sets, was created in 2005.
SAP Hana, an in-memory computing platform combines database, application processing, and integration services on a single platform entered the market in late 2010.
In late 2015, MicroStrategy released MicroStrategy 10.1, introducing many new capabilities including extended support for data preparation and blending, which now empowers a business user with the ability to prepare data directly in the MicroStrategy Desktop or Web product and significantly altered the need for IT involvement or the use of third-party ETL tools.
In the planning stages of a project ensure that all assumptions about a product or solution are validated to make certain that decisions are made based on the most current information. This can especially be an issue if the project selection process to completion is lengthy and during that time period technology, laws or regulation is updated.
As a project progresses incorporating innovation reviews, that include a cross functional team, to determine if any new developments create impacts to the project lifecycle or goals, can help ensure a successful outcome.
This type of review can uncover significant potential impacts such as a mid-project upgrade to a technical component that may eliminate the need for major development or third party integration to create the same feature set or a new law or regulation may limit the ability to use a certain type of consumer or employee data without additional safeguards.
Big Data Project Tip #4 – Leave the legal to the experts
Big Data projects often include large amounts of very sensitive information with many potential people handling and accessing that information. The acquisition, storage, movement, usage and the release of or publication of data is often governed by complex and specific legal agreements, rules, regulations.
For example, if your project includes health information, the Health Insurance Portability and Accountability Act of 1996 (“HIPAA”) and the Standards for Privacy of Individually Identifiable Health Information (“Privacy Rule”) establishes, a set of national standards for the protection of certain health information. (9) These standards address the use and disclosure of individuals’ protected health information by organizations subject to the Privacy Rule. Violations of HIPAA can have big consequences. A Massachusetts hospital, in 2015 paid out $218,000 for permitting employees to use a Web-based file-sharing application to store patients' protected health information. (10)
The inclusion of a project representative focused on legal compliance and governance during planning, monitoring, knowledge transfer and training can help a Big Data project stay compliant with any applicable legal agreements, rules and regulations.
Ensuring that a Big Data project lifecycle contains sign off at key milestone points, by the designated legal representative, is also strongly recommended.
Big Data Project Tip #5 – Security, Security, Security
While security should be an important part of any technology project, Big Data often adds additional security exposure and risk. A Big Data project often has a multiple data sources, many of which may contain sensitive information, storage considerations, several layers of technology, outputs and access points. There is quite a bit to plan for, monitor, control and react to.
Project security responsibilities can vary depending on the industry, size and structure of the organization. In smaller companies it may be an individual project manager’s sole responsibility. In larger organizations there may be policies and procedures created and maintained by divisions under the leadership of a Chief Information Security Officer (CISO), Chief Information Officer (CIO), Human Resources Department or a Project Management Office that define the project manager’s level of responsibility.
Project security on a Big Data project can’t be less than perfect. Ensuring that security considerations and experts are included in the planning, execution and monitoring of a project can help deliver a more secure solution and create a more secure project and operational environment.
Requiring that a Big Data project lifecycle contains sign off at key milestone points, including during technology selection, by the designated security representative is also strongly recommended.
Big Data Project Tip #6 - Don't forget what comes next
The fastest way to a Big Data project being viewed as a failure is to not provide any type of training, knowledge transfer or change management during the project lifecycle to the end users. Actions based on these three words will provide understanding and empowerment to the users and will ensure that the Big Data solution is leveraged as intended and hopefully yield insightful and actionable information and results.
Safe data handling is an important facet for any organization, but to an even greater extent on a Big Data project. It also is important that all team members and users understand what can and can’t be done with the information within or output from a system.
Big Data projects can be a challenge due to expectations, organizational alignment, process change, and willingness of people to accept change. Making certain that there is training, knowledge transfer and change management plans, appropriate documentation and checkpoints will help a business reap the rewards of their investment.
Big Project Tip #7 – Expect dirty data
Big Data projects often includes data from sources that are not transactional or maintained the same ways enterprise financial, human resources or other standard operational systems store their data. In many cases even enterprise level data is dirty due to neglect or inconsistency of input. Data quality issues can severely impact a projects schedule, costs and render outputs incomplete or useless.
An Experian data quality benchmark report found that “92% of organizations suspect their customer and prospect data might be inaccurate in some way” and that “35%, on average, U.S. organizations believe 32% of their data is inaccurate.” (11)
During the planning stages of a project, sample the data to validate what level of cleaning is required. If data is cleansed prior to a project, ensure the process by which the data cleanse was enforced otherwise the problem will reappear.
When planning a Big Data project, include effort to access, plan, clean and test the data and it is best to be conservative with any estimates.
Big Project Tip #8 – Plan for more resources than you think
Big Data projects are so named because they often store and process large amounts of data. With the amount of information available growing at an ever increasing rate, plan for tomorrow.
Companies already have mountains of information at their disposal and as we enter the age of the internet of things (IoT), more and more data will be produced. The internet of things (IoT) is the connection of objects to the internet or any network that can collect and exchange data. In practical terms it can be a household appliance, a vehicle, an industrial sensor, a thermometer, a medical device any object that can produce, collect or communicate data.
Planning for that future is important, as before that Big Data project is live the original technical resource estimates may be far exceeded. Developing a contingency plan or change process at the start of a project for increasing the projects technical resources can help to minimize any impact as well as set expectations.
Conclusion
Having a well-planned Big Data project strategy can help companies reach organizational and project success goals.
Big Data projects often have high reward, but only when they are well conceived, planned and implemented.
Footnotes:
(a) What is a zettabyte? A zettabyte (ZB) is a unit of measure for computer memory or data storage, 1 ZB = 1,000,000,000,000,000,000,000 bytes.
The following chart illustrates the relationship between different storage units.
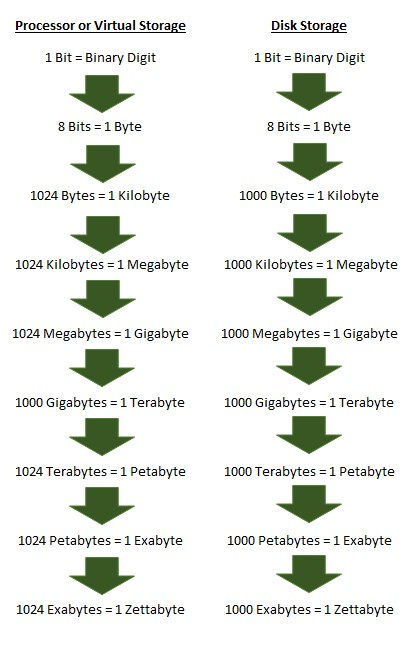
References and Citations:
(1) IDC Online, Press Release, 11/9/2015, http://www.idc.com/getdoc.jsp?containerId=prUS40560115
(2) Department of Health and Human Services, Website, 1/22/2016, http://www.hhs.gov/hipaa/for-professionals/compliance-enforcement/data/complaints-received-by-calendar-year/index.html
(3) Information Security Media Group, Data Breach Today Website, 1/22/2016 http://www.databreachtoday.com/litigation-c-320
(4) Gregory Valyou, Quotation, President of Client Solutions, Rock Pine Partners, 1/22/2016
(5) IBM Website, 1/22/2016, http://www.ibm.com/big-data/us/en/
(6) Microsoft Website, 1/22/2016, https://www.microsoft.com/en-us/server-cloud/solutions/data-warehouse-big-data.aspx
(7) SAP Website, 1/22/2016, http://go.sap.com/solution/big-data.html
(8) Oracle website, 1/22/2016, https://www.oracle.com/big-data/index.html
(9) U.S. Department of Health & Human Services Website, 1/22/2016, “Summary of the HIPAA Privacy Rule”, http://www.hhs.gov/hipaa/for-professionals/privacy/laws-regulations/
(10) Healthcare Finance Website, 1/17/2016, “Massachusetts HIPAA fine shows the financial risk in healthcare breaches”, http://www.healthcarefinancenews.com/news/massachusetts-hipaa-fine-shows-financial-risk-healthcare-breaches
(11) Experian, Data Quality White Paper, January 2015, https://www.edq.com/globalassets/whitepapers/data-quality-benchmark-report.pdf
Disclaimer, Copyright and Trademark Statement
This article is provided for informational and educational purposes. It makes no warranties as to the claims, accuracy or fitness of information provided, referenced or cited. Use of the information, instructions and any examples contained in this work is at your own risk. There should be no implied endorsement of this article by any person or organization referenced.
All trademarks, company, product and services names, images, descriptions, or public website content are property of their respective owner as source referenced. It is your responsibility to ensure that your use thereof complies with such licenses and/or rights.